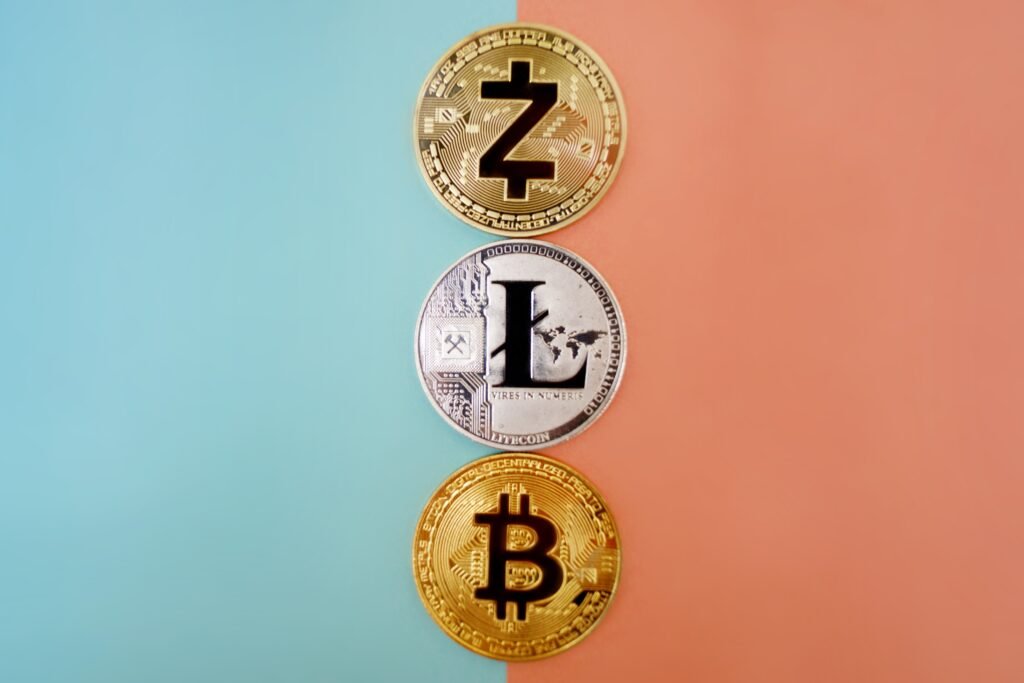
Many advanced crypto trading platforms make use of machine learning techniques to provide advanced trading techniques. These techniques can provide solutions such as answering questions, provides language analysis, and create text. This is a big achievement of deep learning with regards to crypto space. The push money Official website makes use of deep learning techniques on the quant models of cryptocurrencies. This includes techniques such as transformer models and language pretrained. It is the biggest milestone in deep learning, and it is widely used in capital markets too.
The product is created using action research techniques in quantitative finance and explores how transformer models apply to multiple asset classes. Crypto is considered to be the perfect asset class for quant models. This is because these assets have transparency and digital DNA. Quantitative finance has now adapted itself to new technologies and research of deep learning. These ideas of deep learning are emerging and play an important role in crypto scenarios.
- Graph neural networks: datasets of blockchain are unique alpha sources for quant models. The data of blockchain is hierarchical intrinsically, and it can be represented using a graph containing nodes that have addresses connected by edges that represent transactions. The quant models can be used to predict the volatility of bitcoin in the exchange depending upon the characteristics of the addresses that are doing transactions with the exchange. GNNs focus on models that work on graph data structures.
- Generative models: quant models of machine learning cannot work with historical datasets. The crypto trading platform makes use of a predictive model to check the highly volatile pricing of cryptocurrencies. Generative models create synthetic data which matches the training dataset distribution. This technology can be used to create new orders which match the distribution of order books. This is a way to combine synthetic and real datasets to create a large dataset that can train an advanced deep learning model.
- Semi-supervised learning: there is a scarcity of labelled datasets in the crypto industry, and this limits the quant models which can be created in real crypto scenarios. With this feature, it is possible to make price predictions depending on the activity of OTC desks. This deep learning technique works by creating models which can learn with small datasets and a lot of data that has unlabeled data. This concept is similar to a teacher who presents some concepts to a group of students, and others are left for self-study and homework. The labelled dataset can be used to learn about frequency or trade size, while the unlabeled one can be used to expand training.
- Representation learning: selection and feature extraction are important aspects of any learning model of quant machine, and it is relevant in problems that are not properly understood, like crypto-asset predictions. Using this feature, it is possible to make a predictive model for bitcoin pricing by checking order book records. While using this technology, one needs to determine which features or attributes can serve as predictors. This can help in automating the learning of features to build effective models. It does not rely on human feature modelling and can extrapolate features using unlabeled datasets. This is used to predict volatile bitcoin pricing in an automated manner.
- Neural architecture search: this process of creating learning models is highly subjective. A good example of the implementation of this technology is the forecasting of Ethereum price depending upon chosen Defi protocols. Based on the nature of the issue, one can choose some preferences about the architecture and model that should be used. The solution is based on subjective opinions and domain knowledge about the impact of Defi protocols on Ethereum pricing. This technology of machine learning works by automating the generation of models. When a dataset and a target problem is given NAS will evaluate possible architectures of neural network and provide promising results as outputs. The method can be used to process dataset, which includes trading in decentralized exchanges and produce models that can predict pricing of coins and the price change trend based on records.
Apart from these methods, there are numerous deep learning techniques that have an impact on the crypto industry to some extent.